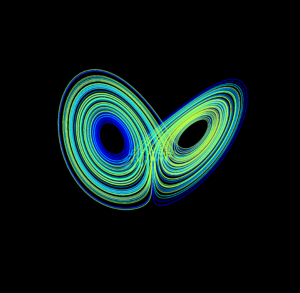
Learning Complex Dynamics
The central focus of this work is to extract predictive models of complex, nonlinear dynamical systems from data. We focus on strategies that enable feature-based representations of complex spatiotemporal processes and techniques that can embed expert and prior physical knowledge. Our aim is to extract model representations that are amenable for planning, control, and ….
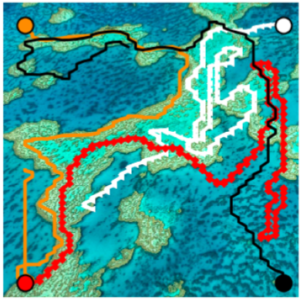
Adaptive Sampling
Being able to estimate and predict information about dynamic processes deepens our understanding of biological, chemical, and physical phenomena in the environment. Often, these dynamic processes exhibit complex, spatio-temporal behaviors. Mobile robots are particularly well-suited to monitor these processes because of their abilities to carry sensors and adapt their sensing …
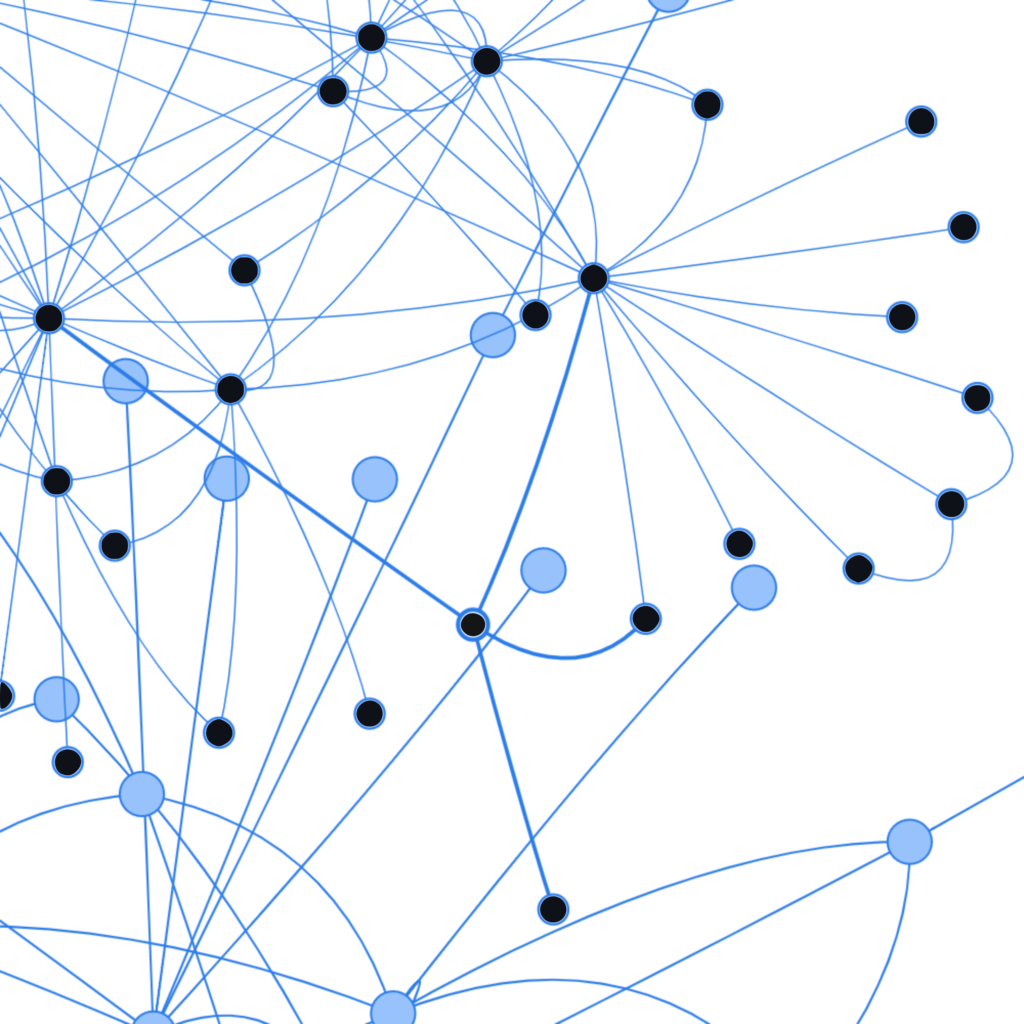
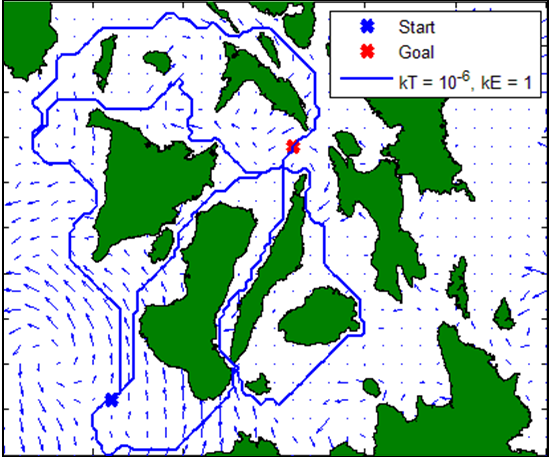